The Role of Attack Surface Hygiene in Protecting Your Large Language Models

Large language models (LLMs) have emerged as powerful tools, revolutionizing industries and enabling organizations to achieve new heights. However, with the increasing sophistication of cyber threats, the security of these AI systems has become a pressing concern. A comprehensive security approach that encompasses proactive measures and continuous vigilance is essential to safeguard your organization’s LLM infrastructure effectively.
The first step towards safeguarding LLMs is understanding the diverse attack surfaces of these systems. This includes recognizing the vulnerabilities inherent in the LLM infrastructure, such as data inputs, APIs, and human interaction elements. Organizations can prioritize their security efforts and implement targeted protective measures by identifying these potential entry points for unauthorized access or manipulation.
The table below outlines various methods and potential attacks that could be directed at AI systems, specifically focusing on language models like ChatGPT. These methods are categorized into different areas, such as prompt injection, training attacks, agent alterations, tools exploitation, storage attacks, and model vulnerabilities. Each category encompasses a range of tactics that could be used to manipulate or harm the system.
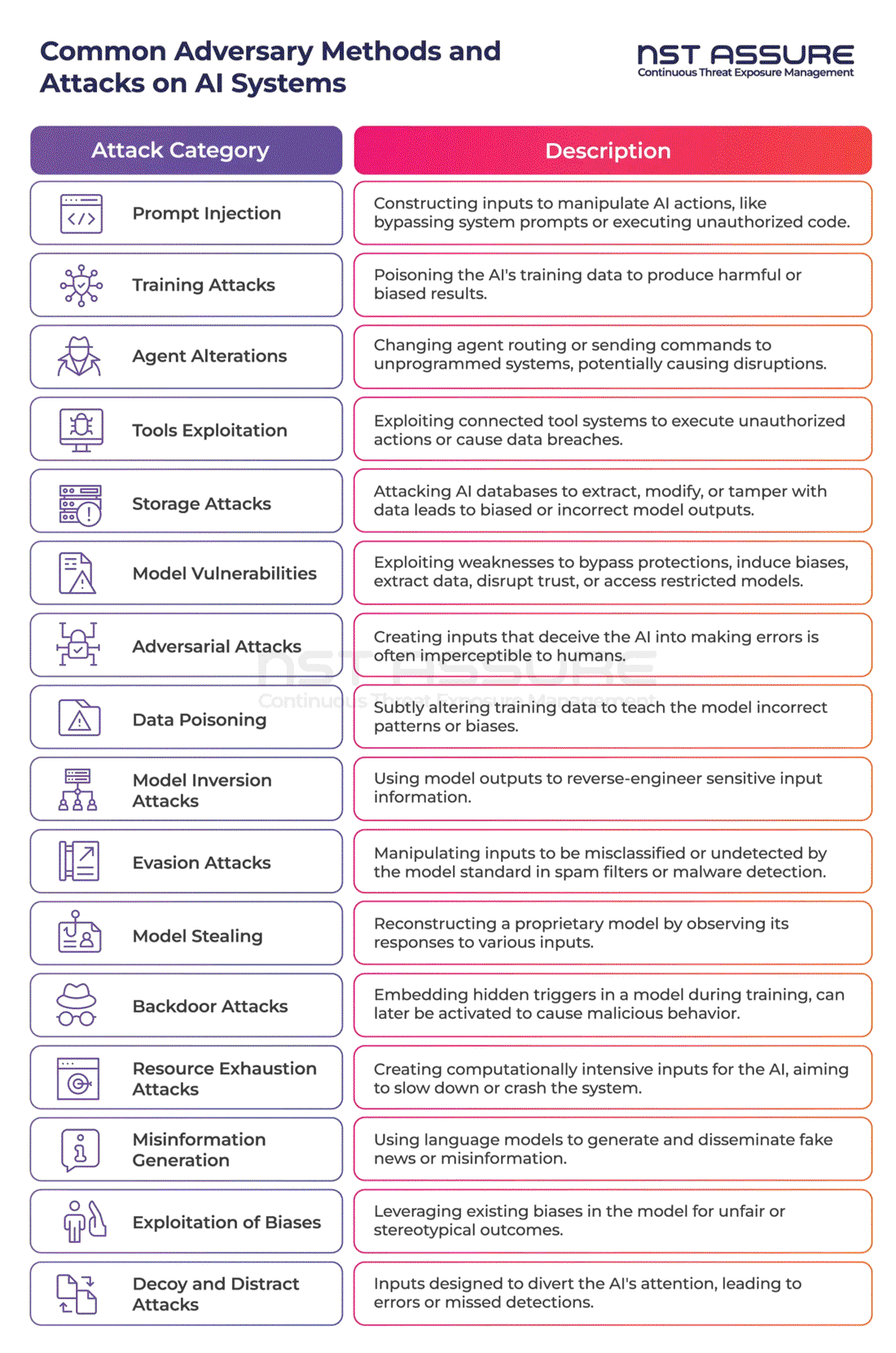
The security of LLMs is not a static endeavor; it requires continuous vigilance and adaptation to evolving threats. Organizations must stay abreast of emerging cyberattacks and adapt their security strategies accordingly. LLMs hold immense potential to transform industries and drive innovation. However, their security must not be taken for granted. By adopting a comprehensive approach that combines proactive security measures with continuous vigilance, organizations can safeguard their LLMs, protect valuable data, and maintain the integrity of their AI-powered operations. Continuous Threat Exposure Management (CTEM) emerges as a critical strategy in this endeavor, providing an essential shield against a diverse range of potential attacks.
Understanding the attack surface of LLMs is critical and it involves identifying vulnerabilities across various components - from data inputs and APIs to human interaction interfaces. Each element, whether a point for prompt injection, a training data source susceptible to poisoning, or a potential backdoor in model training, represents a possible avenue for exploitation. The above outlined table of adversary methods, ranging from training attacks to misinformation generation, underscores the multifaceted nature of these threats.
However, the defense against such threats is not a one-time effort. Continuous Exposure Management implies a perpetual state of vigilance and adaptation. This ongoing process enables organizations to detect and mitigate threats promptly, maintaining the integrity of their LLMs.
Moreover, in a landscape where AI systems like LLMs are increasingly integral to operations, safeguarding them is not just about protecting a piece of technology; it's about preserving the foundation of innovation and trust upon which modern organizations are built. Continuous Threat Exposure Management (CTEM), therefore, is not just a technical necessity; it's a strategic imperative for any organization looking to harness the full potential of LLMs while ensuring their resilience against ever-evolving cyber threats.