How CISOs Can Leverage AI in Cyber Security
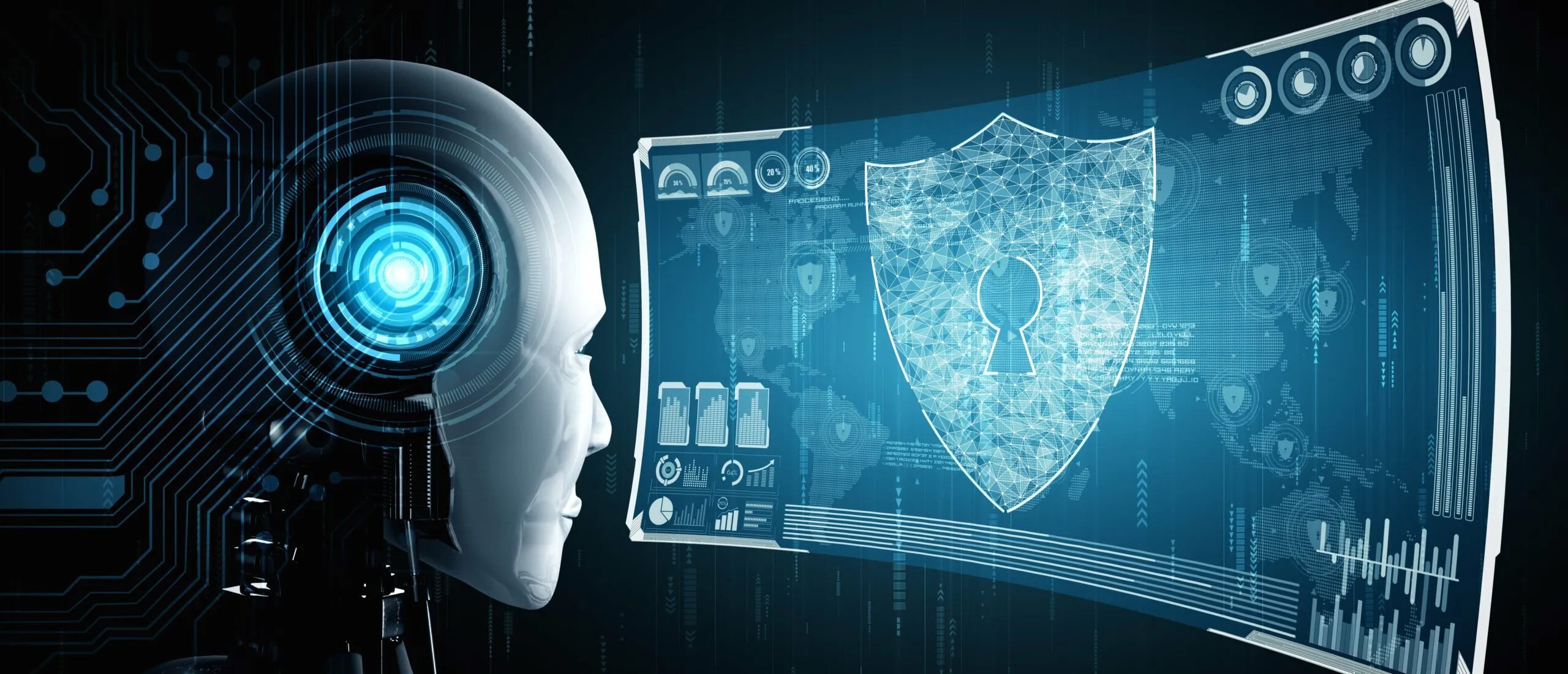
Today’s digital landscapes are rapidly evolving with cyber threats, making advanced defense mechanisms more essential than ever. Artificial Intelligence (AI) has emerged as a pivotal tool for Chief Information Security Officers (CISOs) in bolstering cybersecurity measures, offering capabilities far beyond traditional methods. Recent statistics from the U.S. underscore the escalating cyber threat landscape.
This article will delve into the role of AI in modern cybersecurity, showcase real-world examples of AI thwarting cyber-attacks, and highlight the AI-driven capabilities of NST Assure CTEM.
In 2022 alone, the country reported 1,802 data breaches, affecting a staggering 422 million individuals. A sectoral analysis reveals that industries like healthcare, financial services, and manufacturing are particularly vulnerable. For instance, data breaches in the financial domain nearly doubled from 2020 to 2022. Similarly, the manufacturing sector witnessed a threefold increase in data compromise incidents during the same period.
This escalating threat landscape underscores the importance of real-time threat intelligence. AI, with its ability to process vast amounts of data at unparalleled speeds, offers a promising solution. By leveraging AI, CISOs can ensure that their organizations are not only protected from known threats but are also equipped to tackle new, emerging challenges.

Advantages of AI vs. Traditional Methods
Detect, Prevent and Mitigate Threats
For CISOs, understanding the advantages and limitations between AI-driven systems and traditional methods in cybersecurity is crucial. Here's a comparative breakdown:
- Scalability and Speed:
a. AI-driven systems can analyze vast amounts of data at incredible speeds, allowing for real-time threat detection and response. Machine learning models, once trained, can process data much faster than any human or traditional system.
b. Traditional methods often rely on manual processes or predefined rules, which can be slow and may not scale well with increasing data volumes. - Predictive Analysis:
a. AI-driven systems use algorithms to predict potential threats based on patterns and anomalies in data, even before they manifest. This proactive approach can foresee threats based on historical data and evolving patterns.
b. Traditional methods are typically reactive, responding to threats after they have been identified, often leading to delayed responses. - Continuous Learning:
a. Machine learning, a subset of AI, continuously learns and adapts from new data. As new threats emerge, the system updates itself, ensuring it remains effective against evolving threats.
b. Traditional methods are static in nature, requiring manual updates and rule definitions to handle new threats. - Automated Response:
a. AI-driven systems can initiate automated responses upon detecting threats, such as writing customized proactive continuous security monitoring correlation rules that can be used in any SIEM or SOAR solution, WAF/WAAP policies to defend possible attack attempts, or simple blocking of malicious IP addresses, without human intervention.
b. Traditional methods often require human intervention for threat response, leading to potential delays and windows of vulnerability. - Behavioral Analysis:
a. AI-driven systems can understand 'normal' behavior patterns of a network or system and detect deviations, indicating potential threats. This is especially useful in detecting zero-day vulnerabilities or insider threats.
b. Traditional methods rely on predefined rules or signatures, which might not detect new or unknown threats. - Data Handling Capacity:
a. AI-driven systems are capable of handling and analyzing vast datasets, including big data, making it suitable for large organizations with complex network structures.
b. Traditional methods can be overwhelmed by large data volumes, leading to potential oversight or system slowdowns. - Adaptability:
a. AI-driven systems are inherently adaptable, adjusting to new threat vectors and changing network behaviors.
b. Traditional methods require manual configuration changes to adapt to new threat landscapes, making them less flexible. - Reduced False Positives:
a. By understanding the intricacies of network behavior and user patterns, AI-driven systems can significantly reduce false positives, ensuring that alerts are more accurate.
b. Traditional methods can generate numerous false positives, especially when faced with unfamiliar but benign activities, leading to alert fatigue.
Real-World Examples of AI Thwarting Cyber-Attacks
For CISOs, grasping the practical applications of AI in countering cyber-attacks is essential. The following examples demonstrate the efficacy of AI in cybersecurity scenarios:
- Fighting Spam and Phishing: AI algorithms have been employed to detect and filter out spam and phishing emails. By analyzing patterns, keywords, and sender information, AI can effectively reduce the number of malicious emails that reach the end user.
For example, in early 2022, GOV.UK experienced a spike in spam feedback due to a technical change, with spam constituting up to 12% of total feedback. This spam ranged from fraudulent advertisements and links to inappropriate content to nonsensical combinations of characters. To address this, a multi-disciplinary team at GOV.UK developed a machine learning spam classifier.
This classifier was part of an upgrade to the user feedback pipeline, aiming to quickly deliver critical insights to decision-makers. The machine learning model was trained to predict whether a feedback response was "spam" or "not spam". By using machine learning, the team could automatically filter out tens of thousands of spam responses, ensuring that genuine feedback was not drowned out by the noise of spam. - Surfacing Anomalies: With the digital landscape becoming increasingly complex, traditional security measures often struggle to identify subtle anomalies that could indicate a potential threat. These anomalies can range from unusual user behavior to unexpected data transfers. One of the challenges in cybersecurity is distinguishing between legitimate user behavior and malicious activities.
For instance, a user accessing a database at an unusual time might be working late, or it could be a sign of a compromised account. AI-driven systems can establish a baseline of behavior for users and network traffic. By continuously monitoring and learning from user activities and network events, these systems can detect deviations from the norm.
For example, if an employee who typically accesses the system during regular business hours suddenly starts downloading large amounts of data at midnight, the AI system would flag this as an anomaly. Such real-time anomaly detection allows organizations to respond to potential threats before they escalate.
In another instance, AI systems can monitor website traffic patterns. By understanding the typical user journey on a website, AI can identify unusual patterns that might indicate bot activity. For instance, rapid and repeated attempts to access a login page from different IP addresses in a short span of time might be flagged as a potential brute force attack. AI-driven systems can then take preventive measures, such as temporarily blocking the IP addresses or requiring additional authentication. - Protecting DNS Data: The Domain Name System (DNS) is a foundational component of the internet, translating human-readable domain names into IP addresses. However, it has become a target for cybercriminals aiming to access valuable customer and business information.
Cybercriminals often exploit DNS to conduct attacks, such as DNS poisoning. In a DNS poisoning attack, an attacker intercepts a DNS request and sends a fabricated (poisoned) response to the client, redirecting them to a malicious website. This type of attack poses a significant threat to businesses, with more than 30,000 DNS poisoning attacks occurring daily.
Furthermore, 70% of all cyber-attacks involve the DNS layer. To combat this, AI and machine learning techniques have been employed to analyze trillions of DNS queries. By understanding where malicious actors hide and identifying patterns in their activities, AI can offer protection against these types of attacks. This approach not only helps in detecting and preventing DNS poisoning but also provides insights into other potential threats targeting the DNS. - Automated Malware Detection: Malware attacks have become increasingly sophisticated, posing significant threats to both individuals and organizations. Traditional security measures, such as antivirus software and firewalls, often fall short against advanced malware designed to evade these defenses. The WannaCry ransomware attack in 2017 was a stark reminder of the evolving threat landscape. This ransomware affected over 200,000 computers across 150 countries, targeting systems running the Microsoft Windows operating system. The attack demanded a ransom in bitcoin to restore access, disrupting critical services worldwide.
In response to such sophisticated threats, AI-powered tools have been developed to enhance malware detection processes. These tools analyze vast amounts of data to identify patterns that might be indicative of malware behavior.
For instance, AI algorithms can analyze the behavior of files, detect patterns consistent with malware activities (like accessing sensitive data or modifying system files), and even identify unusual or anomalous behaviors, such as a file trying to access a resource it typically doesn't use. This multi-faceted approach helps in detecting new variants of existing malware, zero-day attacks, and entirely new types of malware that don't have a known signature.
NST Assure CTEM: A CISO's Secret Weapon
Revolutionizing Cyber Defense with AI
In an era where cyber-attacks are increasingly driven by sophisticated algorithms, relying solely on human-centric defense mechanisms is insufficient. NST Assure Continuous Threat Exposure Management (CTEM) platform is uniquely positioned to fill this gap. Built on a cloud-based architecture, it utilizes artificial intelligence (AI) and machine learning (ML) to automate threat detection and response. Unlike traditional security solutions, NST Assure CTEM platform evolves in real-time by learning from a vast array of data, delivering dynamic insights into potential cyber threats, and enhancing organizational resilience.
Below are examples of how NST Assure CTEM system utilizes AI to enhance threat identification, vulnerability prioritization, and cyber defense actions.
- Identification of Potential for Compromise: AI capabilities enable quick discovery of exploitable vulnerabilities, thereby amplifying the predictive nature of the platform's cybersecurity measures.
- Vulnerability Prioritization: Contextual prioritization of the validated vulnerabilities with AI helps optimize decision-making for vulnerability remediation planning.
- Component-Level Vulnerability Analysis: AI performs detailed scrutiny of each software component of external attack surface, providing nuanced insights into various risk factors.
- Rigorous Validation of Threat Surface Observations: AI algorithms sift through and validate collected threat data, assuring a high level of confidence in its accuracy.
- Dynamic Creation of Payloads:Real-time verification payloads are generated through AI, facilitating immediate validation of emerging threats.
Transformation into Intelligent Actionable Insights:
Machine-Readable Cyber Threat Informed Defense (CTID)
NST Assure CTEM platform utilizes advanced AI algorithms to convert threat surface observations into actionable Cyber Threat Informed Defense Intelligence (CTID) bundles. Through the collection and analysis of various threat surface metrics, the AI-driven engine transforms raw data into valuable, actionable insights. These insights are then formulated into CTID, equipping blue teams with enhanced situational awareness and actionable intelligence.
This innovative approach leveraging AI technology ensures that threat assessments are both accurate and timely, enabling security teams to make well-informed decisions. The AI-optimized CTID generated by NST Assure CTEM is designed for seamless integration into existing cybersecurity frameworks. Compatibility extends across multiple types of security gateways, Web Application Firewalls (WAF), Web Application and API Protection (WAAP) solutions, and Security Operations Center (SOC) platforms like Security Information and Event Management (SIEM) and Security Orchestration, Automation, and Response (SOAR) solutions. By incorporating AI-generated CTID into these security elements, blue teams are empowered to monitor, assess, and counteract emerging cyber threats continuously and proactively.
For Chief Information Security Officers (CISOs), NST Assure CTEM offers a well-rounded solution, featuring:
Proactive Threat Management: The AI-driven platform enables a shift from a reactive to a proactive security posture, identifying latent and interlinked risks within an organization's cyber ecosystem.
Enhanced Visibility: Comprehensive monitoring capabilities offer CISOs a panoramic view of their organization's security landscape, ensuring no threats, subtle or glaring, go undetected.
Streamlined Operations: Beyond detection, NST Assure fosters real-time collaboration among security teams, leveraging AI-generated insights for operational efficiency.
As the cyber threat landscape evolves, CISOs are increasingly turning to AI-driven solutions like NST Assure CTEM. The platform embodies the synergy of AI and traditional cybersecurity, setting new benchmarks in proactive threat management and organizational defense.